SPC Explained: 2021 Guide
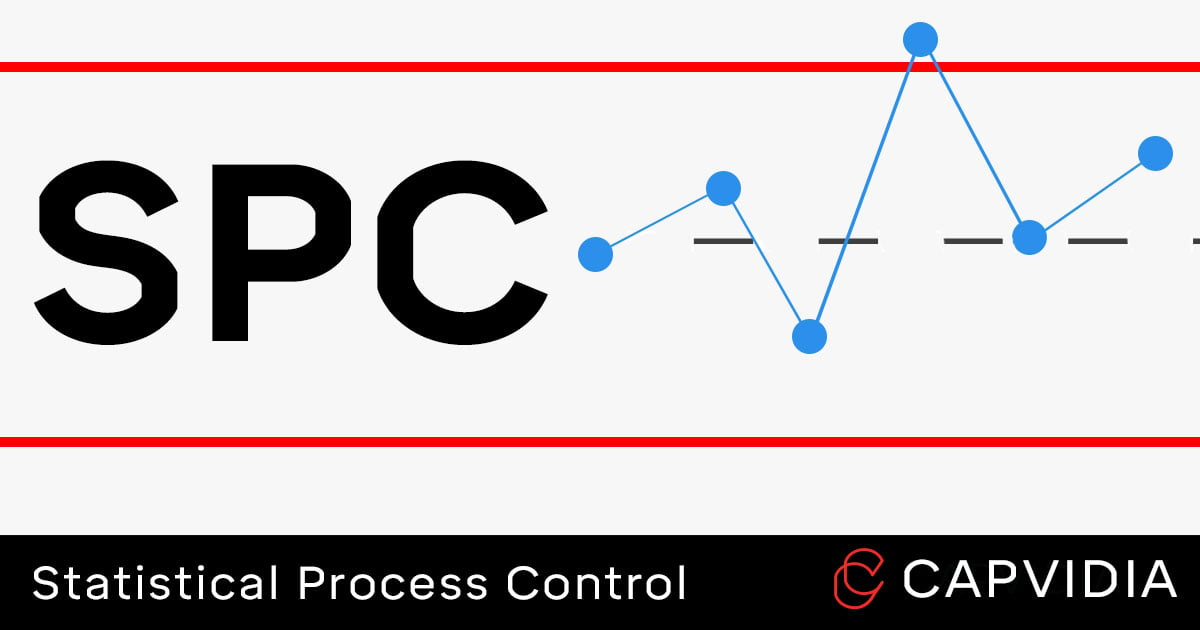
What is SPC?
SPC stands for Statistical Process Control.
In the manufacturing industry, a poor product—defined as not meeting spec— is often the result of a poor process.
SPC is a statistical method of quality control that collects and analyzes data from product and process measurements.
The goal is to determine what falls within vs outside of the process capability leading to preventive or corrective actions when needed.
SPC is part of the five Core Tools for effective quality management with APQP, PPAP, FMEA, and MSA being the other core tools.
Goal of Statical Process Control:
- Understand process and spec limits.
- Ensure a stable process.
- Monitor ongoing process for improvement or corrective action.
Why is SPC important?
In the manufacturing industry, the cost of poor quality (COPQ) measures cost associated with process and product failures.
The best manufacturers have a COPQ score around 1% whereas subpar manufacturers have a score of 5% or higher, which means subpar manufacturers are 5X more likely to pay in scrap, rework, defect, retesting, and recall costs compared to the leaders in the sector.
SPC statistically evaluates a process that is efficient, cost-effective, and able to improve.
Most importantly, it is a method of prevention, which saves more time and money than detecting the problem downstream.
5 Benefits of SPC:
- Prevents Garbage in, garbage out GIGO situation.
- Identifies and reduces processes errors.
- Improves process reliability and quality.
- Prevents non-conforming products
- Better customer satisfaction.
SPC in detail
All manufacturing processes produce variability. It’s a natural part of the process.
The SPC investigates data from process and product measurements to determine whether the variation is acceptable or not.
Types of variation
Variation in manufacturing has two classes:
- Common cause variation: Variation that is normal and part of standard process.
- Special cause variation: Variation that is abnormal and not part of the standard process.
About 85% of variations are common cause, while around 15% are special cause.
But how do we define what is common or special cause variation?
As long as the data is within ±3 standard deviations from the mean, it represents 99.73% of acceptable values (common cause variation). Anything outside the ±3 standard deviations from the mean is unacceptable (special cause variation).
For example, a normal commute to work might take 30 minutes plus/minus 10 minutes.
The 30 minutes is the mean with the ± 10 minutes representing the acceptable deviation range.
If we were to plot a whole month’s worth of commute data, then nearly all data should fall between 20 to 40 minutes for commuting to work aka common cause variation.
However, if there is one day where the commute time was 1 hour and 5 minutes, it’s beyond our acceptable range and we have to find out why. The reason? An accident that created a long traffic jam aka special cause variation.
The goal of the SPC is to eliminate special cause variations.
SPC tools
There are 14 quality control tools used in SPC. Seven are quality control tools and the other seven are supplemental tools.
Quality Control Tools:
- Cause-and-effect diagram
- Check sheet
- Control chart
- Histograms
- Pareto chart
- Scatter diagram
- Stratification
Supplemental Tools:
- Data stratification
- Defect map
- Event logs
- Process flowchart
- Progress center
- Randomization
- Sample size determination
Control chart
The most popular SPC tool is the control chart.
A control chart graphically represents the gathered data with predetermined control limits. Think of the control chart as a bell curve laid sideways in order to see the time sequence of data points.
The control limits, as explained above in the variation section, are ±3 standard deviations from the mean.
Anything that falls within that range between the upper control limit (UCL) and the lower control limit (LCL) is acceptable.
Anything outside that range is unacceptable and defined as special cause variation as shown below.
It’s important to know that control limits are different from specification limits. Control limits represent the voice of the process (what’s feasible) while specification limits represent the voice of the customer (what’s expected). Ideally, control limits should fall within spec limits.
Selection of the right control chart depends on the type of data: variable (continuous) or attribute (discrete). Variable data is preferred when available.
Control charts for variable data:
- Xbar R chart
- Run chart
- XmR chart
- Xbar S chart
- EWMA chart
- Median R chart
Control charts for attribute data:
- P chart
- NP chart
- C chart
- U chart
Understanding the data
After all data is plotted on the control chart, it should fall between the control limits.
Examples of common cause variation include:
- Wear and tear of machines and tools.
- Conditions such as time of day, season, temperature, humidity, etc.
- Change in material properties.
- Variability in operator work.
- Regular measurement variations.
Anything outside the control limits is a special cause and must be addressed.
Examples of special cause variation include:
- Failed controller.
- Incorrect adjustments to equipment.
- Process shift.
- Change in measurement system.
- Malfunctioning machines or broken tools.
- Material property that is out of spec.
- Operator mistake.
However, there can be situations where all data points are within control limits but still potentially be “out of control.”
Common cause variation data points should be within control limits but also randomly plotted. If a discernable pattern can be noticed, then further investigation is needed.
Examples of within control limits but out of control process includes:
- Cyclical effect.
- Excessive variability.
- Lack of variability.
- Alternating values.
- Run.
- Trend.
Two Phases of SPC
- Phase 1: Identify and establish process to eliminate special cause variations.
- Phase 2: Predict future measurements and improve process.
Future of SPC workflows and tools
SPC collects and produces valuable data for immediate but also long-term improvements.
Most data is viewed as pass/fail and often discarded. But in a century of Big Data & AI, data is the new oil.
A model-based definition (MBD) introduces data back into the ecosystem for improved designs, improved parts, and improved operations done quicker, cheaper, and more efficiently.
MBD is a growing industry practice of having the 3D CAD model be the “single source of truth.”
This means the CAD model along with semantic product and manufacturing information (PMI) can make data collection for APQP, MSA, PPAP, and SPC more robust and faster in real-time as the digital thread head towards Industry 4.0 practices.
Most importantly, advanced analytics and deep learning has the power to elevate SPC workflows to the next level as the manufacturing industry leans into digital and data technologies.
MBD Benefits for SPC:
- Automated: No need for human transcription or interpretation.
- Build and optimize a reliable and repeatable process.
- Data mapped back to the single source of truth.
- Start of the digital transformation from design to manufacturing.
- Valuable data to mined for analytics and business insights
Have other questions about statistical process control?
Contact us and we’ll glad to help answer questions about SPC and improving process capability.